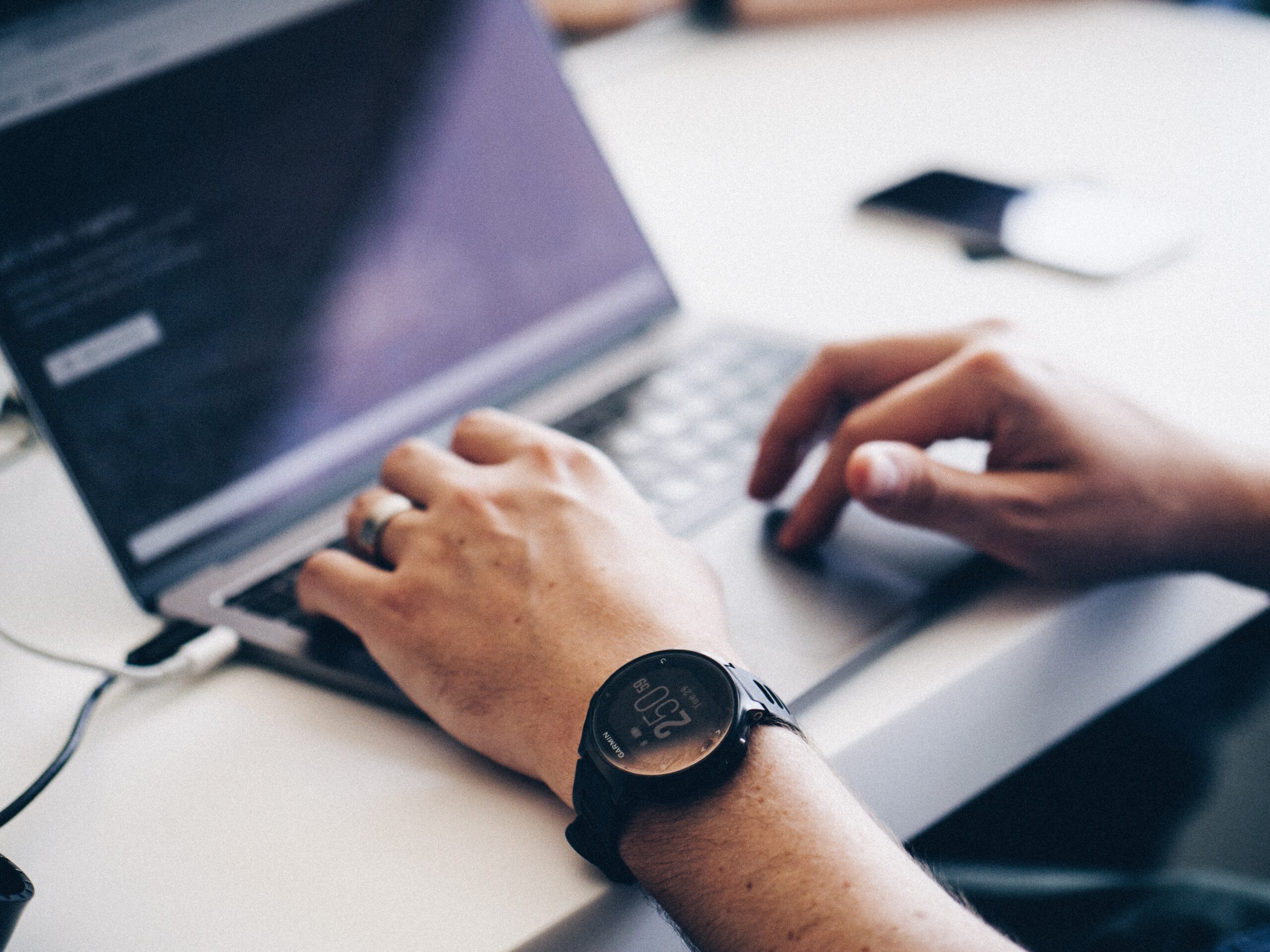
Why MetaMeticulate?
Metadata is vital to keep data flowing. Metadata describes the actual data. What is the content of the dataset? How was the dataset created? What are you allowed to do with the dataset and what not? How can people and machines access the dataset? Searching, finding and using data is made possible by high-quality metadata. Data must be published according to the FAIR data principles: data must be Findable, Accessible, Interoperable and Re-usable. Without good metadata it is not possible to publish data that meets these requirements.
MetaMeticulate explained
The aim of MetaMeticulate – the metadata improvement initiative is to increase the quality of metadata through an iterative do-check-act process. Characteristics describe at a high-level which aspects of metadata quality should be measured. This could, for example, be the FAIR data principles mentioned, or the data maturity model. Metrics act as key performance indicators (KPIs) to measure those characteristics and are used to assign a quality score to a metadata record. Which characteristics and which metrics are important differs from use case to use case.
Workflow
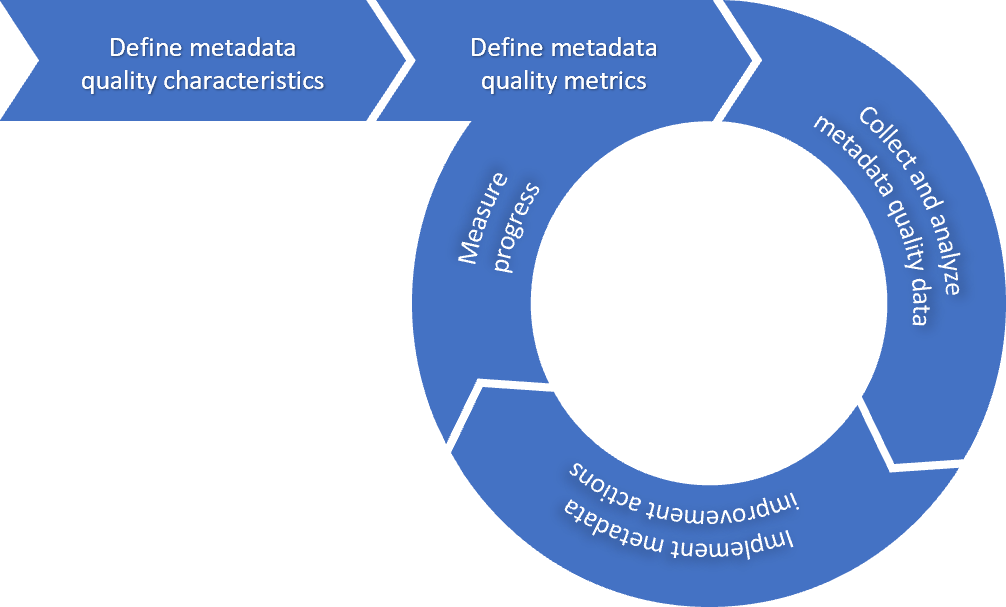
After selecting characteristics and metrics once, the data describing the quality of the metadata records to be evaluated can be collected. Improvement actions can be implemented based on the results. Progress can then be measured, after which a go/no go moment follows: if a metadata record has achieved the required quality level, no further improvement actions are necessary. If not, the cycle is repeated, until the record reaches the required level.
By periodically going through this automated process, the quality of metadata and data is increased. This promotes the reuse of data and supports organizations in taking the next step in data-driven working.
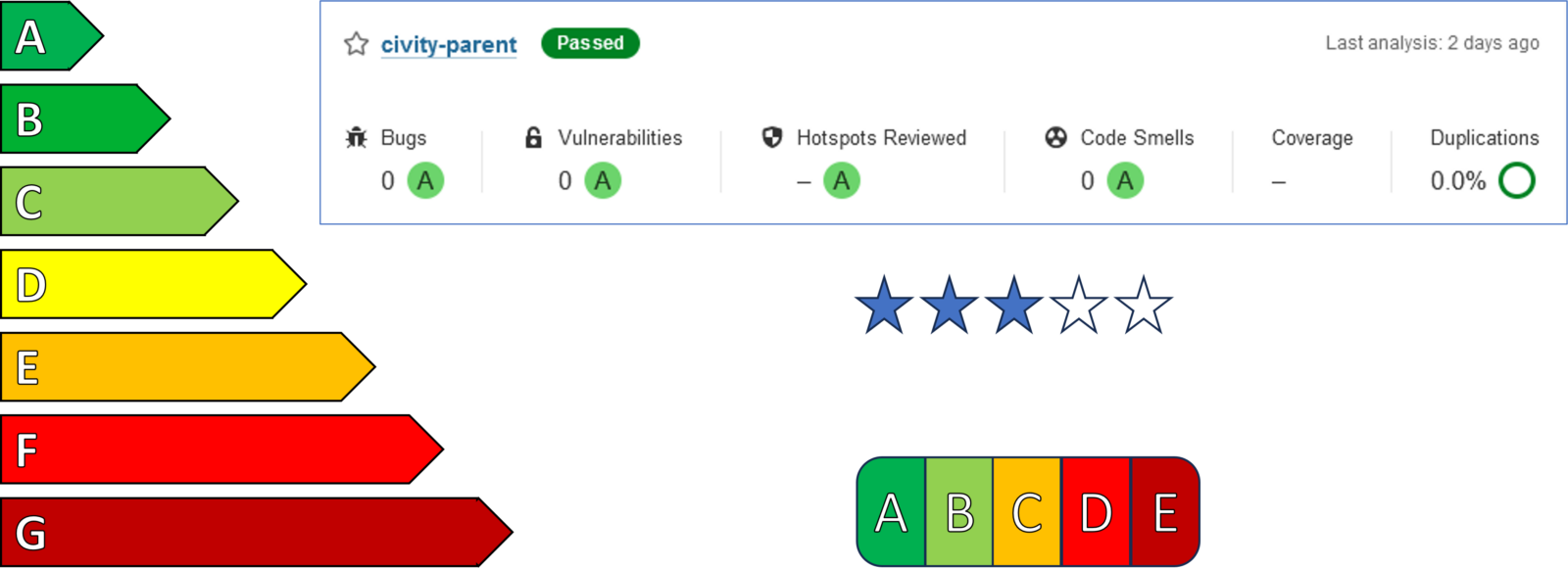
MetaMeticulate workflow: optimizing your metadata quality process
Define metadata quality characteristics
High level characteristics of metadata quality such as FAIR data principals or the data maturity model
Define metadata
quality metrics
Metrics to actually measure metadata quality such as completeness, licensing, or an AI model to evaluate descriptions
Collect and analyze metadata quality data
Automated process to calculate metrics and derive conclusions
Implement metadata improvement actions
Based on outcomes of previous steps decide on what actions to take and execute
Measure progress
Measure progress by comparing to previous iterations using analytical tooling
Decide to iterate again or finish
Go/no-go to decide if a new iteration is needed
MetaMeticulate is an initiative from
<your organization name could be added here too>
MetaMeticulate is supported by
<your organization name could be added here too>
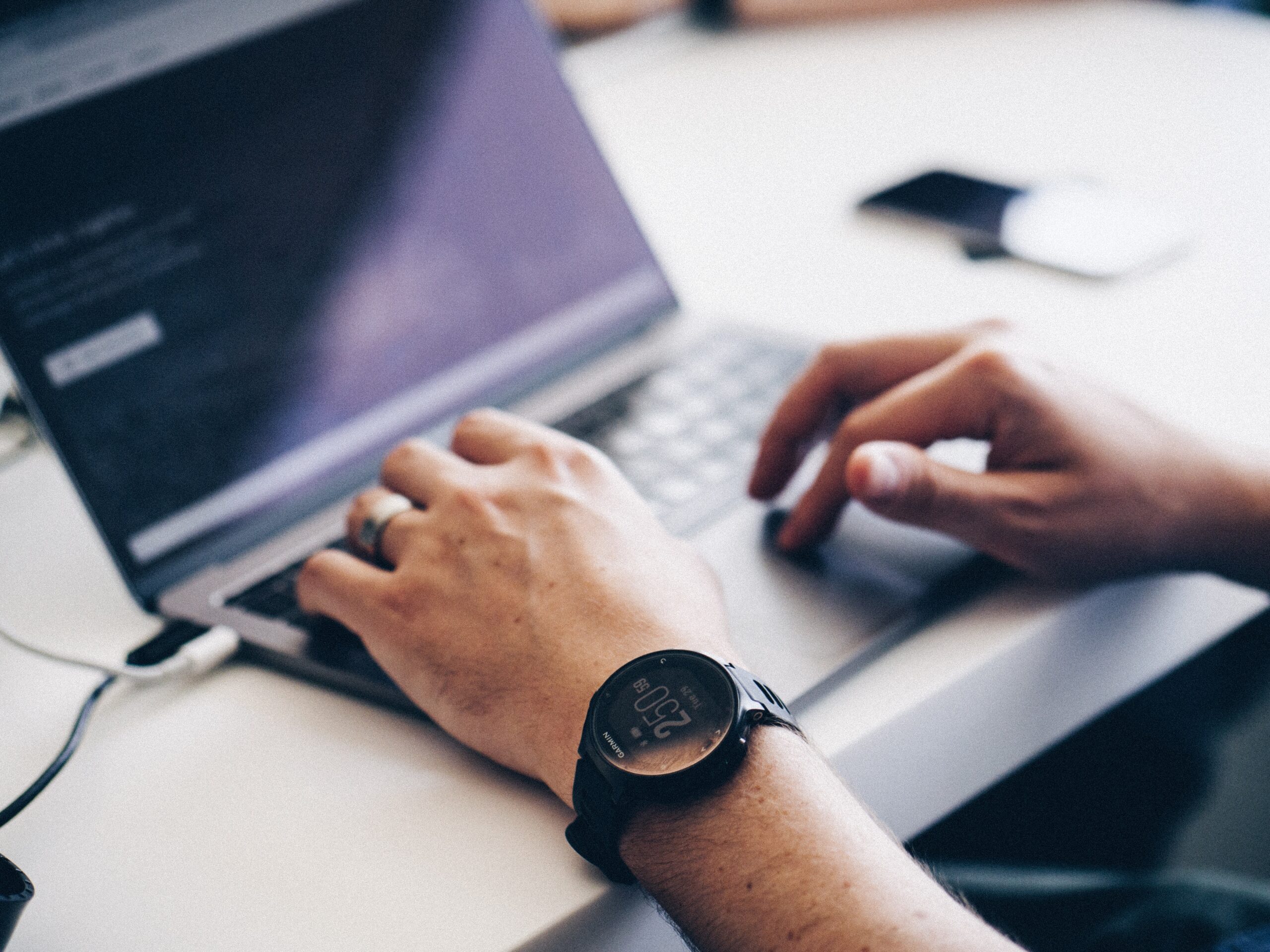
Want to know more?
Contact us by reaching out to us by phone or email if you want us to explain our solution in more detail.